- Everyday Automations
- Posts
- How Can We Mitigate Bias in Generative AI?
How Can We Mitigate Bias in Generative AI?
Generative AI has paved the way for new and innovative technologies, but also brings with it new challenges such as combating inherent bias.
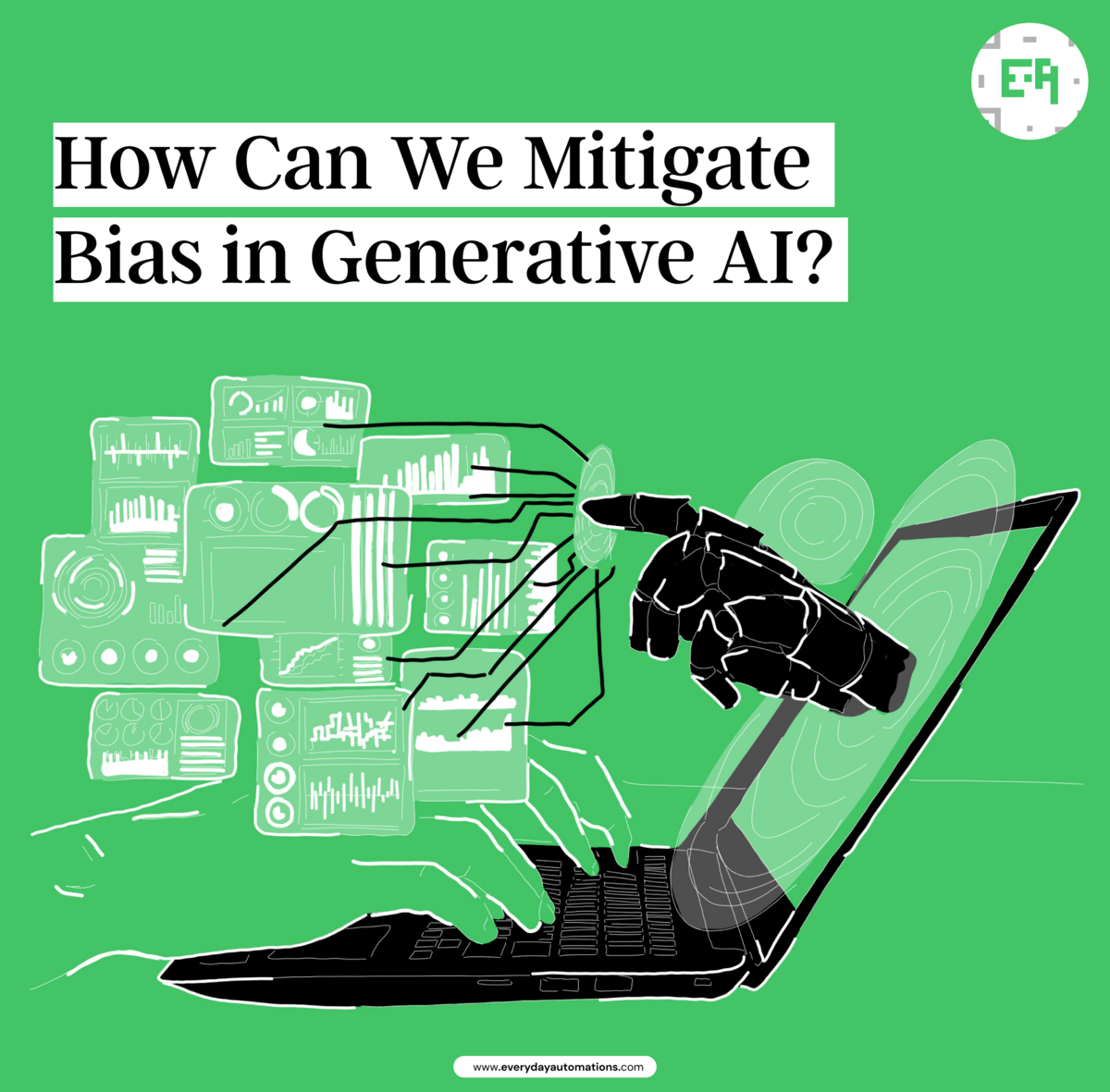
As generative AI has exploded onto the scene with tools like ChatGPT, new challenges are sprouting up alongside. One of these is working to ensure the creation of fair and inclusive AI systems that can remain as neutral and bias-free as possible. AI systems learn from inputs created by humans, and all of us are biased in certain ways and to varying degrees. So in the AI training process, these biases are passed along to the AI system.
What Is Generative AI and Bias?
Generative AI uses algorithms to create realistic-seeming content ranging from text to images to even audio and video.
One example includes art created via human-machine partnership, as in the case of Jason Allen and his AI generative software MidJourney, which created a prize-winning artistic work entitled “Théâtre D’opéra Spatial.” Another example is the fashion industry’s potential use of software called DALL·E, which would take written descriptions of garments and create visual representations.
In certain uses, especially text-generative AIs like ChatGPT, cognitive biases are a big problem. For example, biases in the political arena may include:
Authority bias – an inclination to trust in perceived authority figures
Availability bias – an idea gains credibility from familiarity as it spreads
False consensus – assuming a few loud opinions represent more supporters than there actually are
Dunning-Krugar effect – having confidence in an opinion despite a lack of knowledge
Declinism – romanticizing the past and comparing it to a bleak view of the current times
Framing effect – conclusions based on how the same information is presented
Groupthink – wanting to fit in
Chatbots that continue “learning” as they interact with users can be at a greater risk of adopting these biases than those built in from the beginning.
Identifying and Mitigating Bias
So how do we identify and mitigate bias in generative AI?
It starts with ensuring that inputs (the training data itself) are diversified. When the machine learns from multiple, possibly conflicting sources, we see an improved likelihood of neutral responses or those that present multiple viewpoints to an argument.
On the other side, auditing outputs allows for discovering biases that come through initial machine learning, which can be adjusted with further inputs.
Recently, an AI-generated entertainment production ran into issues when dialogue was generated that was considered hateful toward a demographic of people.
Twitch, the platform where this was aired, had to deal quickly with the apparent bias in AI. The initial response was to blame OpenAI’s Curie model for having undiscovered and unmitigated biases.
The results of generative AI can take on the prejudices, views, perspectives, ideology, moral stances, and inclinations of all groups and individuals contributing information. Policing this is just about as difficult as policing the entire corpus of written content on the internet.
Mitigation
Bias mitigation techniques are currently grouped into three main categories: adversarial training, counterfactual data augmentation, and fair representation learning.
Lassi is a Google-affiliated method for attempting to mitigate biases in high-dimensional data. Developed by three women of color, Lassi stands for “Latent Space Smoothing for Individually Fair Representations” and seeks to ensure fairness and inclusivity within technology and all that is produced by such technology.
An illustration might help here. Earlier, non-generative AI tools tried to solve facial recognition. But as these tools rolled out to law enforcement and others, they didn’t seem to work evenly across all populations. They identified white faces extremely well but had significant difficulty distinguishing between faces of color—even between two faces that any human could easily distinguish.
Why did this happen? It turns out that the AI was fed images of almost exclusively white people. There was bias, perhaps unintentional, built in from the beginning.
Building an ethical accountability framework through which collaboration with field-specific experts can pair with new technological advancements would aid in identifying and mitigating biases in generative AI.
Fast-forward to today. Now we aren’t just using AI to identify patterns in photos. We’re using it to create photos, stories, and narratives. It’s more important than ever to solve this bias equation.
The Future of Generative AI and Bias
The future of AI has great potential for generating the content, exploring new ideas, improving efficiency, reducing costs, and personalizing experiences. But it also has alarming potential for feeding biases, creating and spreading disinformation, and more.
Ensuring that AI pulls from legitimate, balanced sources helps to minimize bias in data and that responses are as neutral as possible. And some organizations are hard at work developing software that mitigates biases. These tools are growing alongside generative AI, helping ensure that the future of AI will keep in mind fairness to all people, transparency in the origination of sources, and accountability wherever possible.
Researchers, policymakers, technology industry leaders, and members of society must work together to push AI forward in fair and ethical ways, reducing bias at the same time.
Reply